Motivation
1.提出一种 cvr 预估中的对比学习方案 CL4CVR, 能够更高效地利用稀疏而宝贵的监督信号
Method
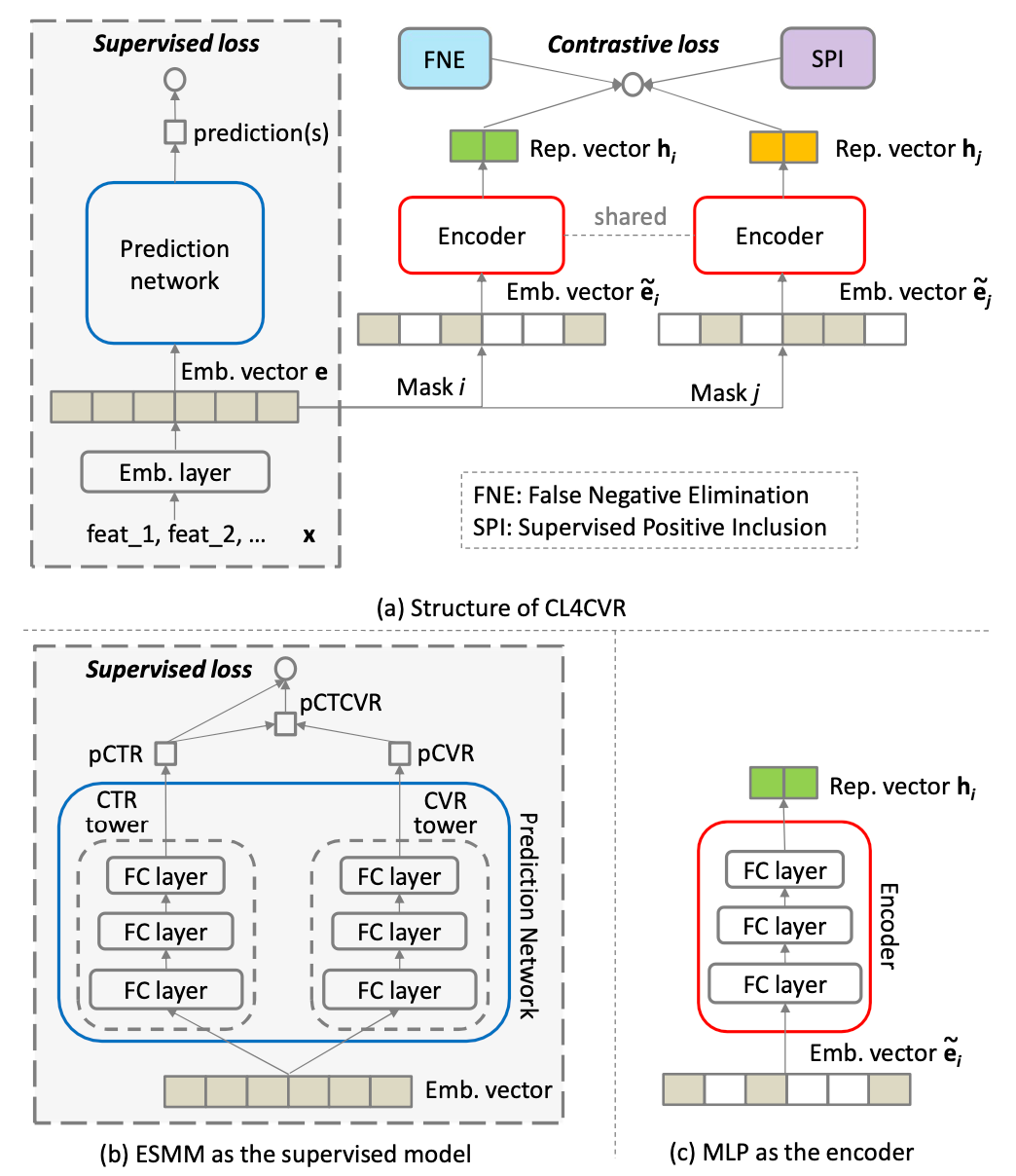
Feature Masking v.s. Embedding masking
intuitively,
1.对比学习的本质是想学出来更好的 presentation: 对于 1 个样本来说, 我们基于它产生 1 个正样本和一堆负样本, 使得原样本和正样本在 embedding 空间的距离拉进, 同时让原样本和其他负样本在 embedding 空间上拉远
2.想采用对比学习, 首先要决定如何产生同一个表达的不同的 view, 本文采用的是 embedding 维度的 masking 的方法
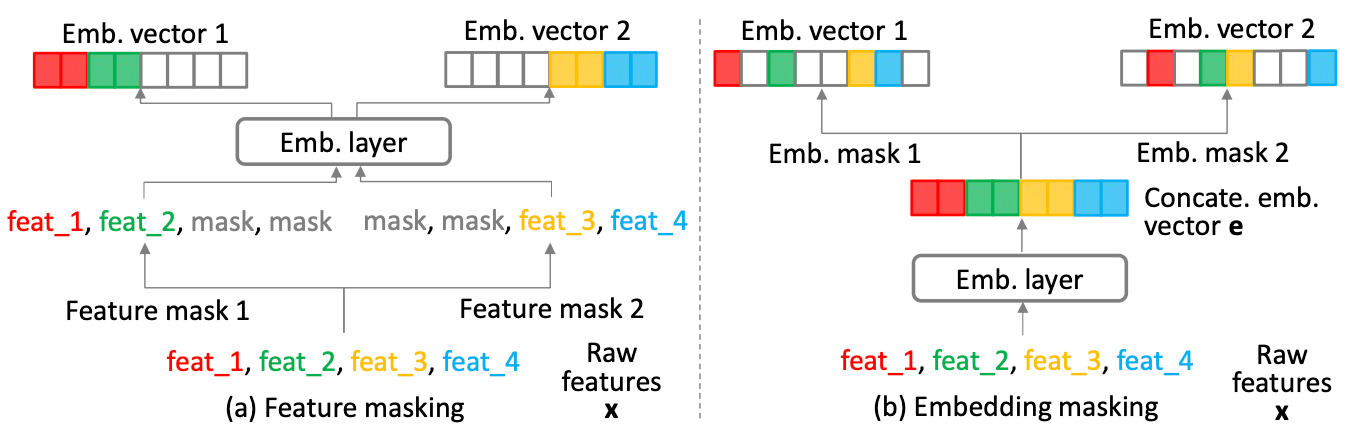
CL4CVR
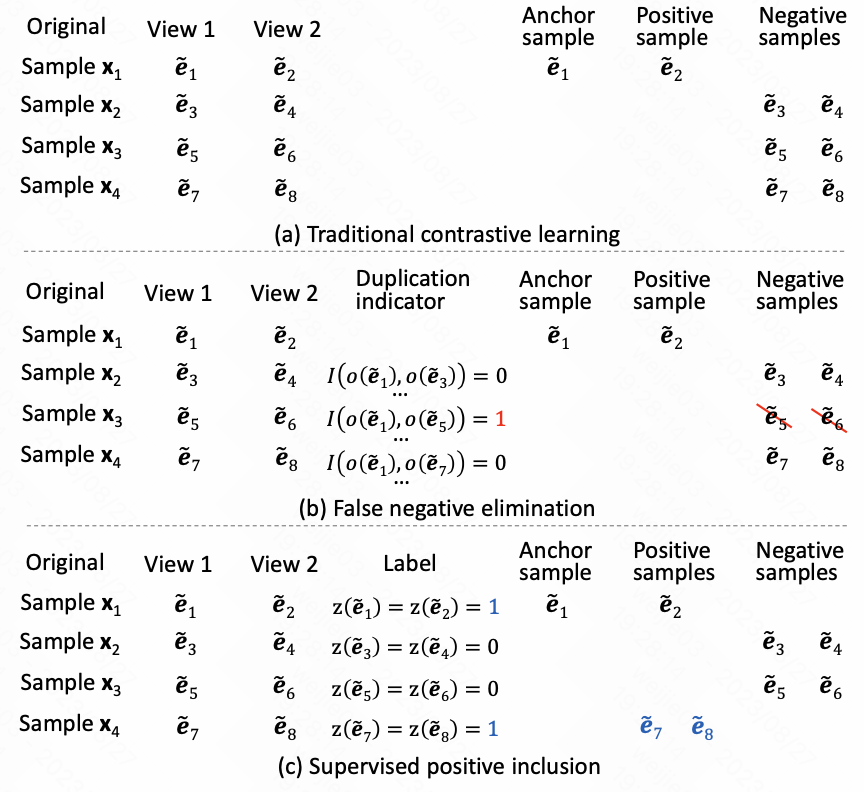
intuitively,
1.当我们产生了不同的 embedding view 之后, 想用在 cvr 预估里面, 要做两个比较 practical 的处理
2.false negative elimination. 在实际我们拿到的训练样本中, 由于延迟转化等问题的存在, 导致 2 条日志组成的两条样本中可能特征完全相同, 这时候我们统一对特征相同的样本做个处理: 只保留特征不同的样本
3.supervised postive inclusion. 这里我们想做的是 supervised 对比学习, 所以我们只对生成的增强数据里面要对根据 label=1 做有监督的对比学习; 给定一个 anchor sample $\tilde e_i$ , 我们产生它的一个正样本集合 $\mathcal S(i)$ , formulation 如下
4.综合如上的处理, 得到最终的 loss
Reference
[1]. Contrastive Learning for Conversion Rate Prediction.
转载请注明来源, from goldandrabbit.github.io