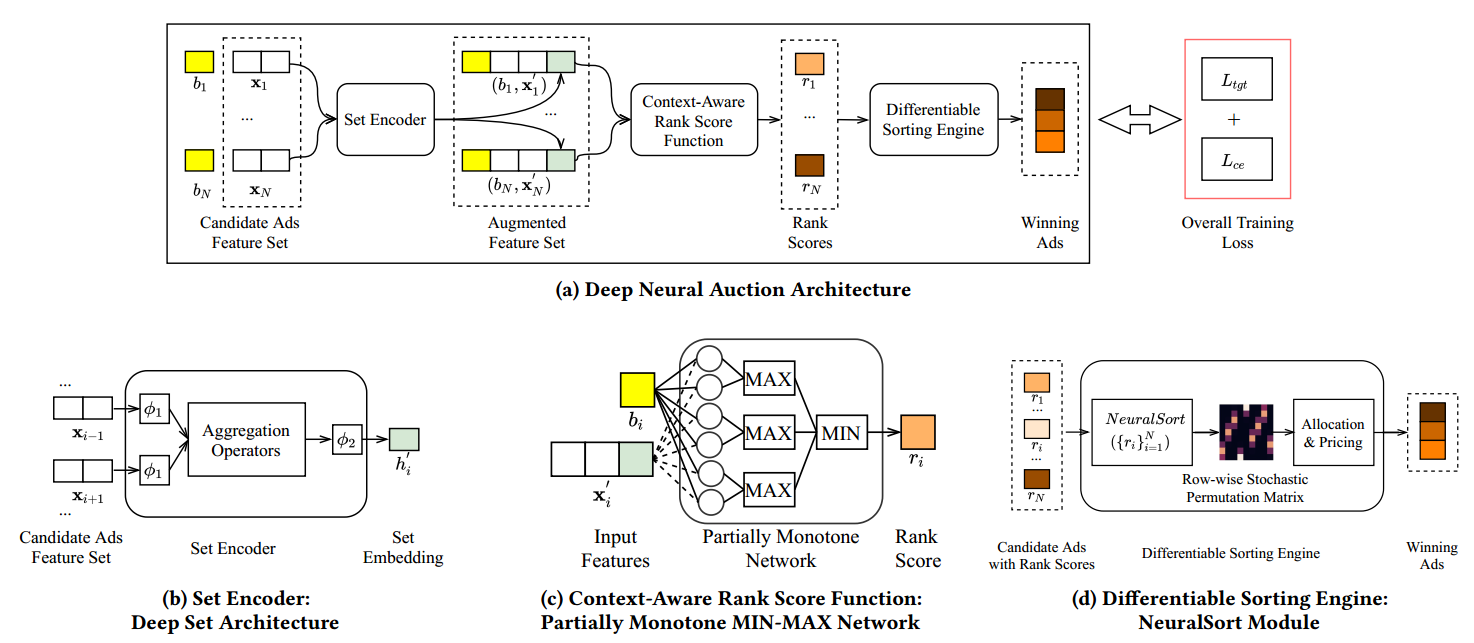
Overview
1.RegretNet: Optimal Auction through Deep Learning 遗憾网络: 通深度学习下的最优拍卖
2.Neural Auction: End2End Auction 端到端的多目标机制学习
3.Context-Intergrated Auction 考虑上下问的拍卖机制
Auction Design Basics
通过 Deep Learning 的方法优化 Mechanism Design, 其中 Auction Design 定义如下
A set of $n$ bidders $N=\left \{1,…,n \right \}$ and $m$ items $M=\left \{1,…,m \right \}$.Each bidder $i$ has a valuation function $v_i: 2^M \to \mathbb R_{\ge 0}$, where $v_i(S)$ denotes how much the bidder values the subset of items $S \subseteq M$.
If a bidder may have additive valuations. In this case she has a value $v_i (\left \{j \right \})$ for each individual item $j\subseteq M$, and her value for a subset of items $S \subseteq M$ is $v_i(S) = \sum_{j\in S}v_i(\left \{j \right \})$.If a bidder’s value for a subset of items $S \subseteq M$ is $v_i(S)={\rm max}_{j\in S} v_i(\left \{j \right \})$, we say this bidder has a unit-demand valuation. We also consider bidders with general combinatorial valuations.
Bidder $i$ ‘s valuation function is drawn independently from a distribution $F_i$ over possible valuation functions $V_i$. We write $v = (v_1,…,v_n)$ for a profile of valuations, and denote $V=\prod_{i=1}^{n} V_i$. The auctioneer knows the distributions $F = (F_1,…,F_n)$, but does not know the bidders’ realized valuation $v$. The bidders report their valuations (perhaps untruthfully), and an auction decides on an allocation of items to the bidders and charges a payment to them.
We denote an auction
as a pair of allocation rules $g_i: V \to 2^M$ and payment rules $p_i: V \to \mathbb R_{\ge 0}$ (these rules can be randomized).Given bids $b = (b_1,…,b_n) \in V$ , the auction computes an allocation $g(b)$ and payments $p(b)$.
A bidder with valuation $v_i$ receives a utility
for a report of bid profile $b$. Let $v_{−i}$ denote the valuation profile $v=(v_1,…,v_n)$ without element $v_i$, similarly for $b_i$, and let $V_i=\prod_{j\ne i} V_j$ denote the possible valuation profiles of bidders other than bidder $i$.
1.An auction is dominant strategy incentive compatible (DSIC) if each bidder’s utility is maximized by reporting truthfully no matter what the other bidders report. In other words,
for every bidder $i$, every valuation $v_i \in V_i$, every bid $b_i \in V_i$, and all bids $b_{−i} \in V_{−i}$ from others.
2.An auction is ex post individually rational (IR) if each bidder receives a non-zero utility, i.e.
for $\forall i \in N, v_i \in V_i$, and $b_{−i} \in V_{−i}$.
In a DSIC auction, it is in the best interest of each bidder to report truthfully, and so the revenue on valuation profile $v$ is
Optimal auction design seeks to identify a DSIC auction that maximizes expected revenue.
Formulation as a Learning Problem
given a parametric class of auctions $(g^w, p^w) \in \mathcal M$, for parameter $w \in \mathbb R^d$ for some $d \in \mathbb N$, and sample a bidder valuation profiles $\mathcal S=\left \{v^{(1)},…,v^{(L)} \right \}$ drawn i.i.d from $F$. The goal is to find an auction that minimizes the negated, expected revenue
RegretNet
RegretNet replace IC constraints with a differenctiable approximation and lifts the IC constraints into the objective by augmenting the objective with a term that account for the extent to which the IC constraints are vialated.
RegretNet measure the extent to which an auction violates the incentive compatibility through the following notion of ex post regret.
Fixing the bids of others, the ex post regret for a bidder is the maximum increase in her utility, considering all possible non-truthful bids. For mechanisms $(g^w, p^w)$, RegretNet is interested in expected ex post regret for bidder $i$:
where the expectation is over $v \sim F$, and $u_i^w(v_i;b)=v_i(g_i^w(b))-p_i^w(b)$ for model parameters $w$
Neural Auction
Model
Multi-objective Modeling
Feature Design
Permutaion-Inequivalent Modeling
Context-Intergrated Auction
Reference
[1]. Optimal Auctions through Deep Learning. Paul Dütting et al.
[2]. Neural Auction: End-to-End Learning of Auction Mechanisms for E-Commerce Advertising https://arxiv.org/abs/2106.03593. Xiangyu Liu et al.
[3]. Neural Auction: 电商广告中的端到端机制优化方法 https://zhuanlan.zhihu.com/p/412872425. Xiangyu Liu et al.
转载请注明来源, from goldandrabbit.github.io