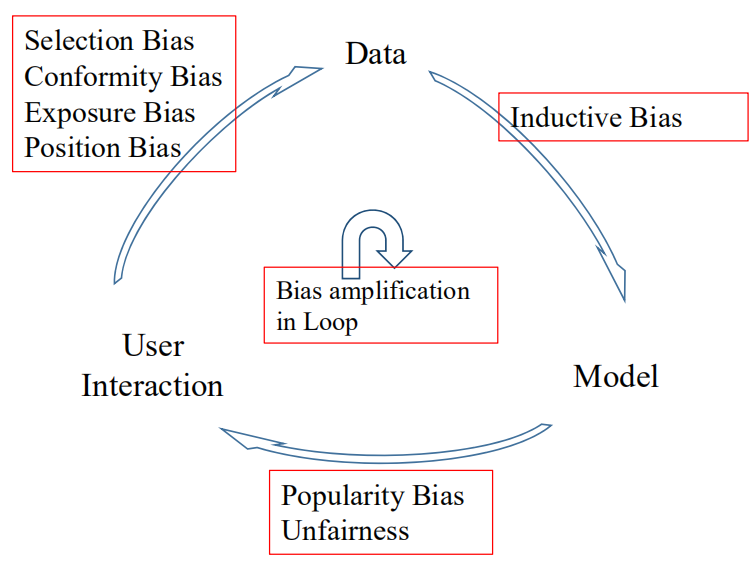
Overview
推荐系统中bias的综述, 对 bias 的分类和产生原因给出了标准化的定义.
Bias in explicit feedback data
Selection Bias. Users are free to choose which items to rate, so that the observed ratings are not a representative sample of all ratings. In other words, the rating data is often missing not at random (MNAR). 用 mt 评论数据训练模型, 点了 4 家外卖都可以给好评, 但是可能只给其中1家点了好评.
Conformity Bias. Users tend to rate similarly to the others in a group, even if doing so goes against their own judgment, making the rating values do not always signify user true preference. dy短视频评论区100个人里面98个人在讽刺/谩骂/反对, 看到这些评论, 第101个用户能否真正客观评论?
Bias in implicit feedback data
Exposure Bias. Users are only exposed to a part of specific items so that unobserved interactions do not always represent negative preference. 在已有的猜你喜欢的内容推荐中, 有交互的item都是被算法推荐的, 并不代表没给推荐的就不喜欢.
Position Bias. Position bias happens as users tend to interact with items in higher position of the recommendation list regardless of the items’ actual relevance so that the interacted items might not be highly relevant. 即使是在同一个推荐列表里面, 排序较高的对相对排序较低的具有更高的点击优先权. 用户的兴趣item/相关item也有可能在排序较低的位置.
Bias in model
Inductive Bias. Inductive bias denotes the assumptions made by the model to better learn the target function and to generalize beyond training data.
Bias and Unfairness in Results
Popularity Bias. Popular items are recommended even more frequently than their popularity would warrant (保证). 热门商品比其流行程度推荐地更高频.
Unfairness. The system systematically and unfairly discriminates against certain individuals or groups of individuals in favor others.不公平性: 对于某个具体的个人偏好不公平.
Reference
[1]. Bias and Debias in Recommender System: A Survey and Future Directions. Jiawei Chen et al. https://arxiv.org/abs/2009.03240v2.
转载请注明来源 goldandrabbit.github.io