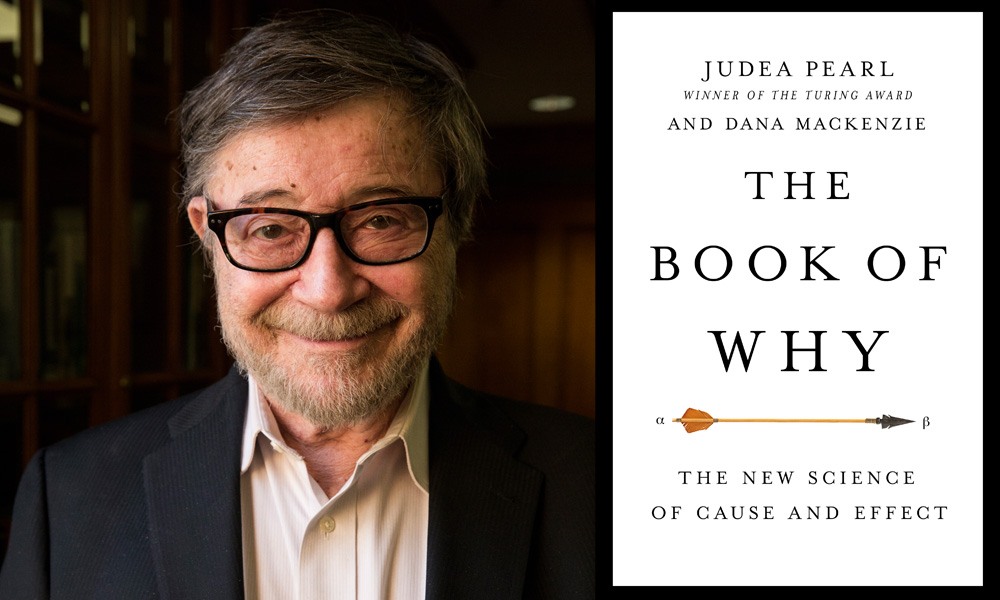
Overview
1.为什么读这本书?
2.Core Idea: 你比你的数据更聪明
3.The Ladder of Causation 因果之梯
为什么读这本书
1.2018 年腾讯笔试题有一道主观题-阐述对于因果关系的理解, 且题面明确提示: 要区别于相关关系, 当时我没有想清楚二者的联系和区别, 后来也没有完全想清楚其中的联系. 直到发现这个问题并非一个简单的问题, 且有着相当长的历史(贯穿了统计学发展史),本书对于这个问题有着非常详尽的论述, 有醍醐惯性之感.
2.本书作者本身因 causal inference 的相关研究获得图灵奖, 是具有统计学背景的计算机科学家的著作, 具备较高权威性.
Core Idea: 你比你的数据更聪明
If I could sum up the message of this book in one pithy phrase, it would be that you are smarter than your data. Data do not understand causes and effects; humans do.
I hope that the new science of causal inference will enable us to better understand how we do it, because there is no better way to understand ourselves than by emulating ourselves.
In the age of computers, this new understanding also brings with it the prospect of amplifying our innate abilities so that we can make better sense of data, be it big or small.
intuitively,
1.因果推断这个新兴学科能够让我们更好地理解我们 (人类) 是如何做到因果推断的.
2.一句话概括本书主要思想: 你比你的数据更聪明. 数据不理解因果, 而人类理解因果.
3.我们处于计算时代, 我们对于因果推断的理解可以增强我们对于因果关系的直觉, 从而让我们更好地读懂数据 (无论是大数据还是小数据).
Original story
圣经里面有一段话
When God finds Adam hiding in the garden, he asks “Have you eaten from the tree which I forbade you?
And Adam answers, “The woman you gave me for a companion, she gave me fruit from the tree and I ate.
“What is this you have done?” God asks Eve.
She replies, “The serpent deceived me, and I ate.”
Here is the point I had missed before: God asked “what” and they answered “why”. God asked forthe facts, and they replied with explanations.
上帝问的是”什么”, 他们回答的是”为什么”; 上帝问的是”事实”, 他们回答的是”理由”.
Moreover, both were thoroughly convinced that naming causes would somehow paint their actions in a different light.
他们 (潜意识里) 深信, 列举原因可以以某种方式美化他们的行为.
Where did they get this idea? For me, these nuances carried three profound implications.
First, very early in our evolution, we humans realized that the world is not made up only of dry facts (what we might call data today); rather, these facts are glued together by an intricate web of cause-effect relationships.
人类进化早期就意识到世界并非由枯燥的事实构成的(我们今天可能称之为数据), 这些事实是通过错中复杂的的因果关系网络融合在一起的.
Second, causal explanations, not dry facts, make up the bulk of our knowledge, and should be the cornerstone of machine intelligence. 因果解释, 而非枯燥的事实本身, 组成了我们大部分的知识, 它应该成为机器智能的基石.
Finally, our transition from processors of data to makers of explanations was not gradual; it was a leap that required an external push from an uncommon fruit.
This matched perfectly with what I had observed theoretically in the Ladder of Causation: No machine can derive explanations from raw data.
It needs a push.
从数据处理者到因果解释者的过度不是渐进的, 而是一次”跳跃”. 借助的是某种奇异的外部能力, 这与我在因果关系之梯上的理论观察完全吻合, 没有那台机器可以从原始数据中获得解释,对数据的解释需要借助外部推力.
The Ladder of Causation 因果之梯
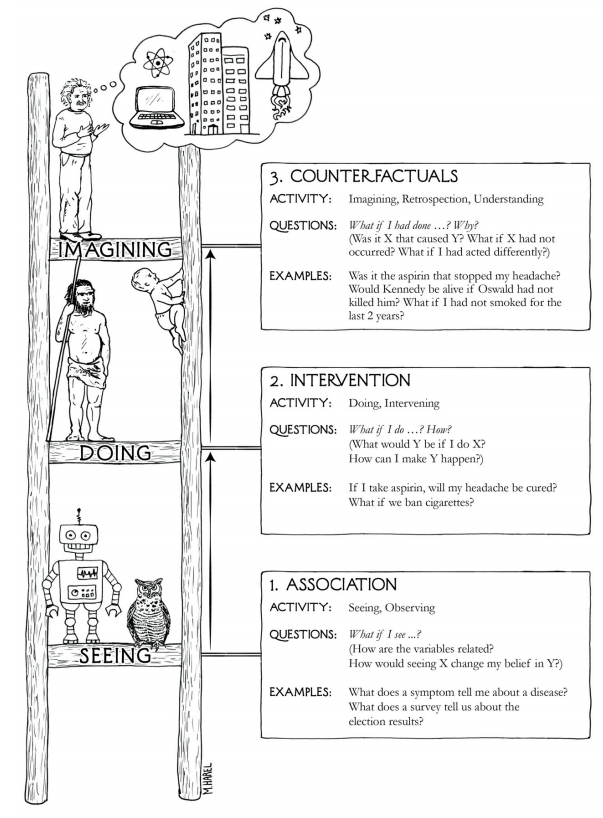
So far I may have given the impression that the ability to organize our knowledge of the world into causes and effects was monolithic and acquired all at once.
In fact, my research on machine learning has taught me that a causal learner must master at least three distinct levels of cognitive ability:seeing, doing, and imagining. 这种能力其实是三种能力: 认知能力, 行动能力, 想象能力.
The first, seeing or observing, entails detection of regularities in our environment and is shared by many animals as well as early humans before the Cognitive Revolution.
The second, doing, entails predicting the effect(s) of deliberate alterations of the environment and choosing among these alterations to produce a desired outcome. Only a small handful of species have demonstrated elements of this skill.
Use of tools, provided it is intentional and not just accidental or copied from ancestors, could be taken as a sign of reaching this second level.
Yet even tool users do not necessarily possess a “theory” of their tool that tells them why it works and what to do when it doesn’t.
For that, you need to have achieved a level of understanding that permits imagining.
It was primarily this third level that prepared us for further revolutions in agriculture and science and led to a sudden and drastic change in our species’ impact on the planet.
intuitively,
1.总结了三层因果结构
第一层
典型问题: 如果观察到…会怎样? “What if I see …?”
典型例子: 购买牙膏的顾客同时购买牙线的概率有多大?
公式化: P(牙线|牙膏)
第二层
典型问题: 如果观察到…会怎样? “What if I see …?”
典型例子:
公式化: P(牙线|do(牙膏))
第三层
典型问题: 假如我当时做了…会怎样? “What if I see …?”
example: 假如我们把牙膏的价格提高一倍, 那么买了牙膏的顾客仍然选择购买的概率是多少?
Reference
[1]. The book of why.
转载请注明来源, from goldandrabbit.github.io